In this talk, Marco Gori introduce the notion of developmental agents, that are based on the theory of “learning from constraints” (see e.g. http://videolectures.net/marco_gori/).
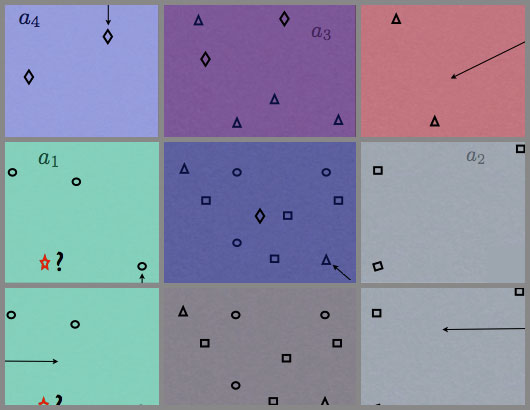
Perceptual and logic constraints
It is claimed that in most interesting tasks, learning from constraints naturally leads to “deep architectures”, that emerge when following the developmental principle of focusing attention on “easy constraints”, at each stage. Interestingly, this suggests that stage-based learning, as discussed in developmental psychology, might not be primarily the outcome of biology, but it could be instead the consequence of optimization principles and complexity issues that hold regardless of the “body”.
In the second part of the talk, he gives insights on the adoption of the proposed framework in computer vision. The proposed functional approach leads naturally to develop different notions of features, the lower level of which are somehow related to classical SIFT. It is pointed out that the adoption of information-theoretic principles are at the basis of the feature generation either at low or high level of the vision computer hierarchy. The functions that are developed are inherently independent of roto-translations and do acquire scale invariance by the minimization of an appropriate entropy-based measure, that is also at the basis of the focus of attention.
Finally, he gives an overview of different constraints emerging at different layers of the hierarchy, and claim that the overall system is expected to work in any visual environment by acting continuously, with no separation between learning and scene interpretation.