Last year I presented the work we at the Computer Vision Center (CVC) did on semantic image segmentation in context of the PASCAL 2009 Visual Object Classification (VOC) challenge. This year, we again fielded teams in several competitions of the PASCAL 2010 VOC challenge.
In this talk, I will discuss the extensions we have made to our approach to semantic image segmentation. I will show how the results of object detectors and spatial priors can be naturally integrated into our hierarchical conditional random field (HCRF) approach based on the harmony potential. The addition of these extra cues, as well as class-specific normalization of classifier outputs, significantly improves segmentation quality.
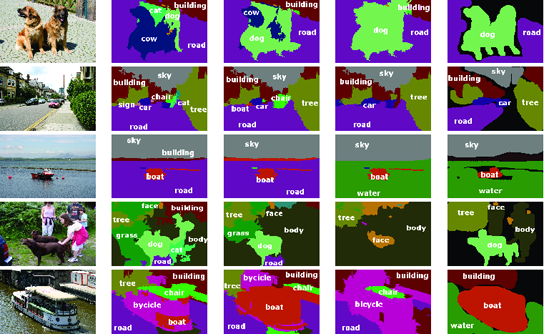
Semantic segmentation in still images
I will also discuss our approach to human action recognition in still images. Action recognition from still images is a new, “taster” competition in this year’s VOC competition. It requires participants to identify the action being performed in individual images and the task is further complicated by the lack of large quantities of training data. Our approach is based on a spatial pyramids over a classical
bag-of-visual-words approach with extensive, class-specific cross validation used for feature selection.
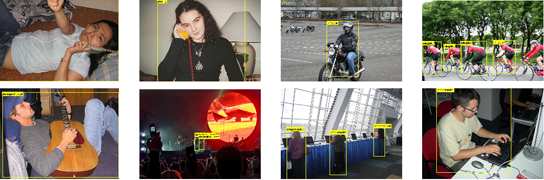
Human action recognition in still images
Our results on semantic object class segmentation show that our approach obtains state-of-the-art results on three challenging datasets: PASCAL VOC 2009, PASCAL VOC 2010 and MSRC-21. In the PASCAL 2010 challenge, our approach won eleven gold medals, taking first place in the segmentation challenge. In action classification, our approach won three gold medals and jointly won the first place award along with INRIA LEAR and University of Surrey.